09
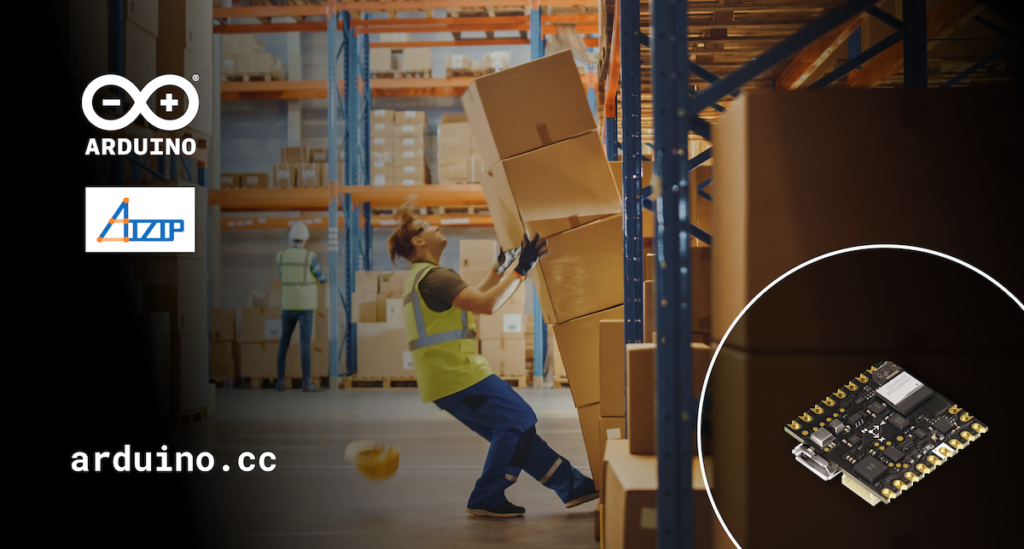
The challenge
Personal safety is a growing concern in a variety of settings: from high-risk jobs where HSE managers must guarantee workers’ security to the increasingly common work and study choices that drive family and friends far apart, sometimes leading to more isolated lives. In all of these situations, having a system capable of sensing and automatically contacting help in case of emergency can not only give people peace of mind, but save lives.
A particularly interesting case – as the world population ages – regards the increasing number of elderly people who are still healthy enough to be independent yet must also accept the fact their bodies are becoming weaker and their bones more fragile. This specific target is more prone to falls, which can result in fractures, head injuries, and other serious accidents that can severely impact the quality of life. Detecting falls early can allow for prompt medical attention and prevent serious consequences. Additionally, detecting falls can help identify underlying health issues or environmental factors that may be contributing to accidents, allowing for appropriate interventions to be put in place to avoid future falls.
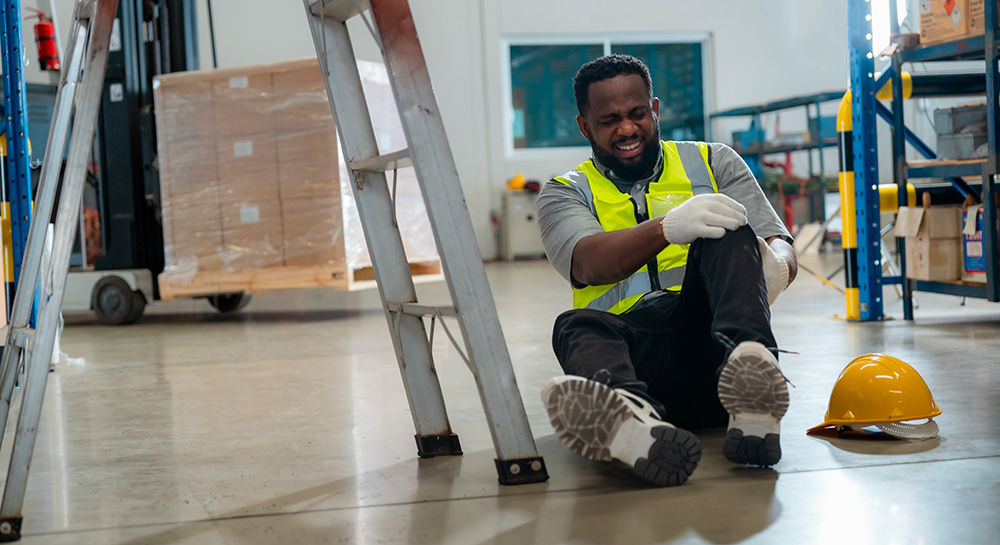
A variety of person-down systems and fall detection methods exist, ranging from threshold-based algorithms to traditional machine-learning applications. The biggest challenge they all share is they suffer from high false-positive triggers. In other words, they cause unnecessary alarm and distress to both the seniors and their caregivers, resulting in unwarranted actions.
Our solution
A tiny but mighty deployment device: Nicla Sense ME
For its project, Aizip selected the Nicla Sense ME: a compact module integrating multiple cutting-edge sensors from Bosch Sensortec, enabling sensor fusion applications directly at the edge. Additionally, the module houses an Arm® Cortex®-M4 microcontroller (nRF52832) leveraging Bluetooth® 4.2. Aizip’s neural network model fits right in with the remaining resources of the microcontroller, thanks to its compact footprint. The result? A small and lightweight device that can be clipped onto one’s belt and worn all day without hassle, able to monitor health parameters and immediately alert assistance in case of fall, with near-zero latency and full respect for privacy.
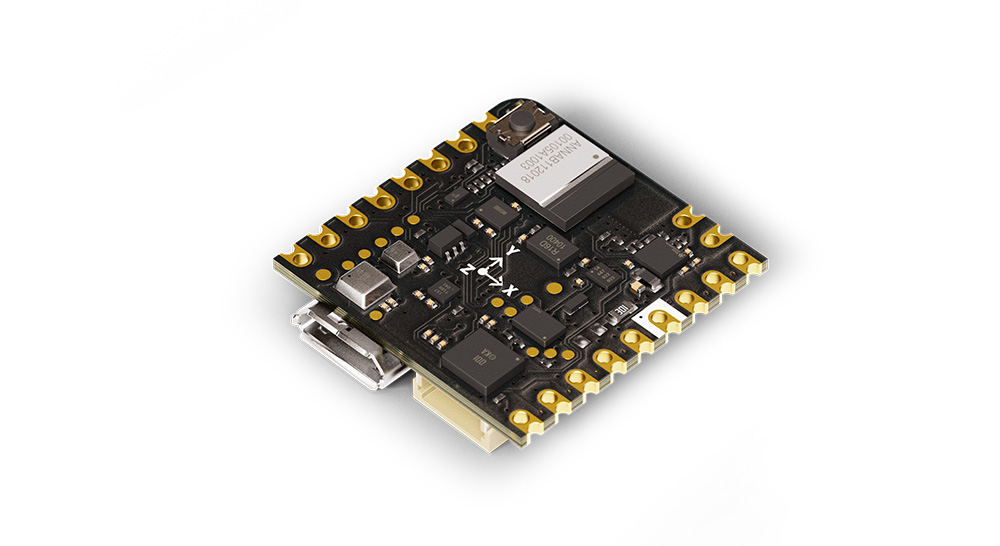
A more accurate fall detection algorithm
Aizip’s fall detection solution integrates a neural network algorithm with sensor fusion to greatly enhance detection accuracy, while also being lightweight enough it can run in real time on a microcontroller. The neural network within the microcontroller continuously processes sensor readings from the accelerometer (BHI260AP) and the pressure sensor (BMP390). Upon detecting a fall, the device sends an alarm via Bluetooth and activates an on-board LED. In order to minimize frequent false alarms that could significantly affect user experience, the neural network is optimized to differentiate real falls from abrupt movements such as jumping, sprinting, and quickly sitting down. The neural network-based algorithm excels at capturing subtle features in inputs, leading to a substantial reduction in false alarm rates compared to threshold-based approaches or traditional machine learning algorithms.
Typical neural networks offer superior performances but also pose additional challenges, when deploying them onto resource-constrained microcontroller devices, due to the extensive computing and memory resources required. The simultaneous need for Bluetooth connectivity and sensor fusion further compounds this issue. However, Aizip’s proprietary efficient neural network architecture makes this solution stand out because it minimizes resource requirements while maintaining high accuracy. The neural network is quantized to 8bit and deployed onto the microcontroller using Aizip’s automated design tool. The implemented model achieves a 94% fall detection accuracy and a <0.1% false positive rate, all while utilizing less than 3KB of RAM. A perfect fit for the low-consumption Nicla Sense ME!
Solving it with Arduino Pro
Now let’s explore how we could put all of this together and what we would need for deployment both in terms of hardware and software stack. The Arduino Pro ecosystem is the latest generation of Arduino solutions bringing users the simplicity of integration and scalable, secure, professionally supported services.
Hardware requirements
- Arduino Nicla Sense ME
- Single-cell 3.7 V Li-Po or Li-Ion battery
- Jumper wires (for connecting the board and the battery)
Software requirements
Conclusion
When personal safety is a concern, smart wearables that leverage AI can help. And processing the data required to monitor health conditions and prevent falls doesn’t have to come at the expense of comfort or privacy: thanks to extremely efficient models like Aizip’s and compact yet high-performance modules like Arduino Pro’s Nicla Sense ME, you can create a discreet and reliable solution able to immediately call for help when needed (and only when needed).
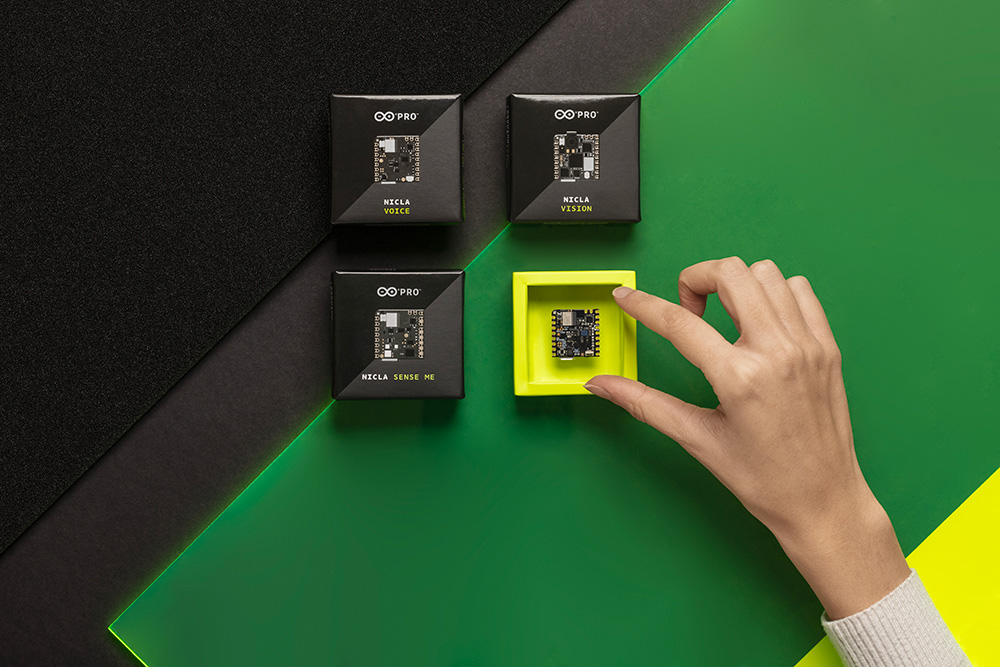
The post Fall detection system with Nicla Sense ME appeared first on Arduino Blog.